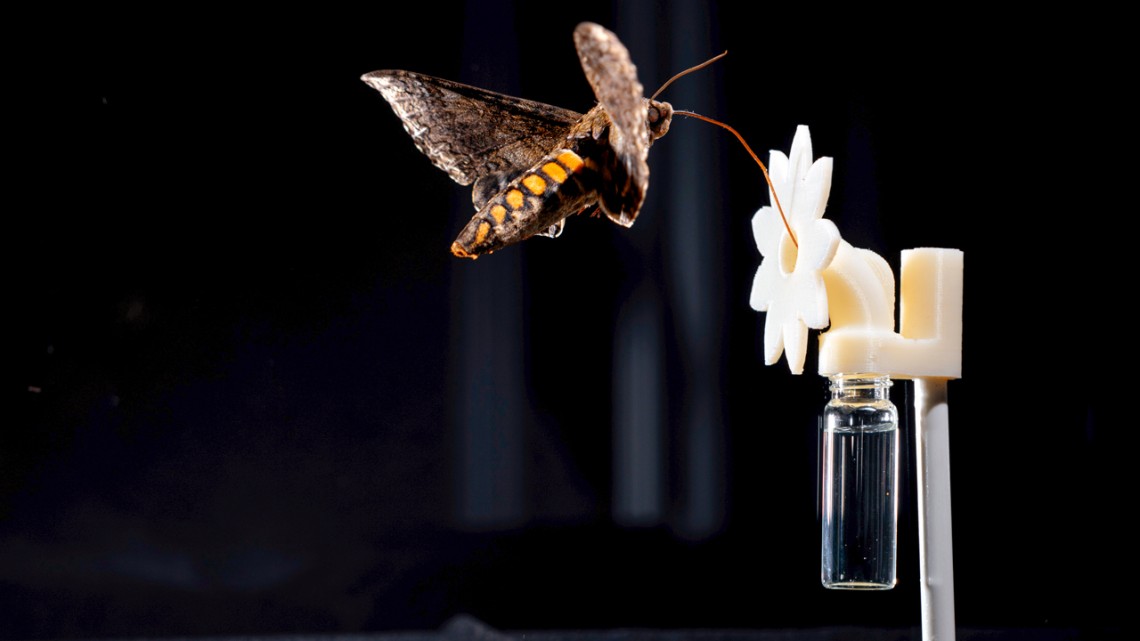
Manduca sexta hovering to feed from a flower, which requires precision and agility.
Study explores moth brains to improve drone flight
By Melanie Lefkowitz
Insects’ brains are small and limited. Yet they fly with speed, grace and accuracy, responding quickly to changing conditions and zooming away from predators.
Thanks to recent advances in fields including computational neuroscience and machine learning, researchers are now studying these neurological systems to develop the next generation of smart, agile and highly adaptive autonomous aerial drones.
Silvia Ferrari, the John Brancaccio Professor of Mechanical and Aerospace Engineering, has been awarded a $400,000 grant from the Air Force Office of Scientific Research to study the brains of moths with the goal of improving drone flight.
“We’re interested in understanding how the brain and sensory modal system of these small fliers function, with very basic principles,” Ferrari said. “And hopefully we can translate some of these principles to aerial vehicles.”
With Simon Sponberg, assistant professor of physics at Georgia Tech, Ferrari will examine the mathematical underpinnings of “spike trains” – the sequences of firing neurons that help the brain learn.
“Spike trains are a very efficient way to carry information, process information and use that information for reasoning and solving problems, so they’re believed to be one of the main reasons the brain is so powerful,” Ferrari said. “But what’s not known precisely is, what are the mathematical principles in which these neurons encode information during these spikes?”
Using control theory, Ferrari’s area of research, she and Sponberg are examining how spike trains help moths hover – for example, to feed from flowers.
“It’s a very precise problem,” she said. “They have to track the flowers precisely, but the flowers may be moving in the wind, or there may be gusts of wind that disturb the flight of the moth, and if a predator arrives the moth has to rapidly leave.”
In related work, Ferrari is collaborating with researchers from Georgia Tech and Carnegie Mellon University on a $1.8 million grant from Georgia Tech to develop a method of combining information from disparate sources, including artificial intelligence algorithms, to help autonomous vehicles effectively process information.
Different methods of gathering information about a vehicle’s surroundings are often based on different principles and can produce data in completely different forms. This makes it hard to synthesize information from multiple sources. It’s even more complicated when trying to incorporate data from machine learning or AI algorithms, Ferrari said.
“Aircraft and spacecraft already have a lot of autonomous functions,” Ferrari said. “Now that people are developing so many new algorithms for computer vision and machine learning, there are many different methods being used for processing sensing data.”
Using an approach developed in Ferrari’s lab, the researchers aim to combine data from the various sources – including AI – into a single, optimized framework.
“There are other techniques for distributed systems,” Ferrari said, “but the key of this work is to include AI and machine learning algorithms that are not often treated in the existing methods, because they have completely different principles and assumptions.”
The researchers hope to test their technique on autonomous vehicles in both virtual and physical experiments.
Media Contact
Get Cornell news delivered right to your inbox.
Subscribe