Nate Silver offers tips to improve predicting power
By Mikhail Yakhnis
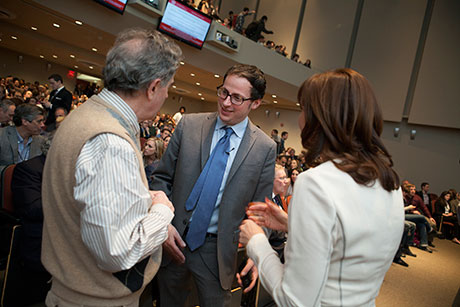
Over the past decade, we have mispredicted earthquakes, flu rates and even terrorist attacks. Yet we seem to have access to more data and computing power than ever.
“Why isn’t big data producing big progress?” asked author, blogger and statistician Nate Silver during his talk, “Big Data: Powerful Predictions Through Data Analytics,” April 5 on campus. Known for his innovative analyses of political polling, Silver is author of “The Signal and the Noise: Why So Many Predictions Fail – but Some Don’t” and author of the New York Times political blog FiveThirtyEight. Silver first gained national attention during the 2008 presidential election, when he correctly predicted the results of the primaries and the presidential winner in 49 states.
“By some estimates, 90 percent of the information in the world has been created in the last 10 years,” Silver pointed out. Yet “The most recent Congress has been one of the most polarized in history,” Silver said. “It has also been the least productive.”
Handling big data more effectively is not necessarily straightforward. With so many inputs, it becomes exponentially difficult to find relationships among the variables. For instance, given five inputs, there are 10 relationships to test for. However, given a set of economic data with 65,000 variables, the number of possible relationships balloons to 1.86 billion.
Even though we are doing a better job of understanding causality, he said, this understanding is lagging behind the number of tests that are being run. As a result, “the gap between what we think we know and what we actually know is increasing,” Silver said, “and that’s really dangerous because we’re human beings and that leads us to make stupid decisions sometimes.”
Silver recommended that we think more “probabilistically.”
“It used to be that we could only predict the cone of uncertainty [of landfall] for hurricanes within 350 miles … now we can predict it within 100 miles,” Silver noted. This comes largely from improvements in statistical methods and computing power.
Secondly, he said, we need to identify our own biases. As an example, he discussed two HR managers – one who works for a company that says it has no bias and one that says it might have some bias. Presented with virtually identical resumes except for whether the prospective employees have a male or female name, the HR manager who claims to have no bias ended up overwhelmingly choosing the resumes from males.
Finally, Silver said to “try and err. Sometimes just measuring things can be quite valuable.” Actually sitting down and getting things on paper can be a useful starting tool, he said.
Silver explained that big data is not going to absolve us of having to be smart and work hard. However, Silver concluded by saying, “If you are willing to test your beliefs by making actual, verifiable claims … and if you have a careful way of weighing new evidence, you will eventually converge toward the truth.”
The talk was part of the Survey Research Institute Speaker Series.
Mikhail Yakhnis '14 is a writer intern for the Cornell Chronicle.
Media Contact
Get Cornell news delivered right to your inbox.
Subscribe