Ride-sharing study findings are scalable to different cities
By Tom Fleischman
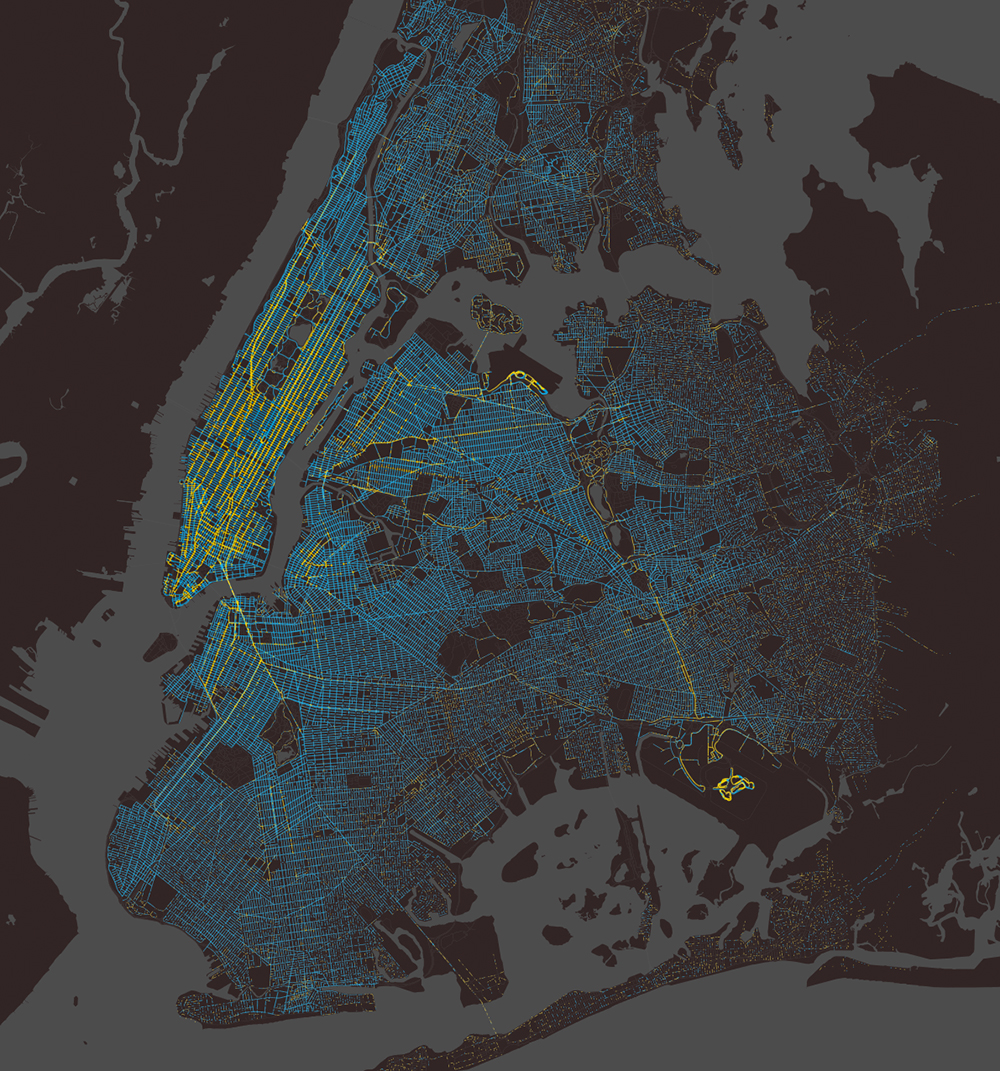
Three years ago, Steven Strogatz, the Jacob Gould Schurman Professor of Applied Mathematics and Stephen H. Weiss Presidential Fellow, helped a group from the Massachusetts Institute of Technology identify the “shareability” of cab service in New York City.
The group found that, indeed, the vast majority of the city’s 150 million taxi trips per year were shareable, and even by sharing a fraction of those rides, pollution and congestion would be significantly lessened. So the obvious follow-up question was: Will that result generalize?
In other words, could a result in a city as densely populated as New York be replicated in different kinds of cities – say, in Vienna? Strogatz and his MIT colleagues asked that very question in their most recent study, and the results could perhaps point city planners toward a “greener” future.
Strogatz – author of four books on mathematics, including “The Joy of X: A Guided Tour of Math, From One to Infinity” – was a key contributor to “Scaling Law of Urban Ride Sharing,” which was published online March 6 in Scientific Reports. Lead author Remi Tachet and principal investigator Carlo Ratti are both at MIT’s Senseable City Lab, which conducted the study.
The researchers analyzed huge data sets of GPS information from four cities (New York [Manhattan only], San Francisco, Singapore and Vienna) then plotted a “shareability curve” for each city as a function of taxi trips per hour. For all cities, the shareability rises quickly as the available trips per hour increases – known as fast saturation – then levels off at a certain point.
These numbers varied greatly for the cities studied, so to generate a shareability index that can apply to both more- and less-dense metropolitan areas, the group applied a natural rescaling law.
The trips-per-hour variable was replaced by the factor L, which represented the ratio between two time scales: the sharing delay (how many minutes you would be willing to add to your trip to share the ride, which the group set at five minutes) and the typical wait time for a cab to arrive. Factored in is the average daily traffic speed in the city, as well as its geographical area.
“This is a dimensionless way of asking, how dense is the taxi availability?” Strogatz said. “Put it this way: You want a taxi right now, but you can’t have it right now. But how long are you willing to wait for that, compared to how long a delay are you willing to tolerate when ride sharing takes you out of your way? That ratio is what the L is measuring.”
In plotting each city’s shareability curve as a function of L, instead of its own unique trips per hour, the study reveals that all four cities (as well as a theoretical prediction) fall along basically the same line. Key to the study, Strogatz said, is the fact that it includes no adjustable parameters – simply determining L for each city gives the model strong predictive power.
“It’s a good theory in that it totally sticks its neck out,” Strogatz said. “It’s saying, ‘The world is like this, or it’s not.’ And as it turns out, the world is pretty darn close to what the theory says.”
The plots of the four cities suggest that there is a “sweet spot,” Strogatz said, where the number of available vehicles would be optimal for maximum sharing and minimal congestion.
The researchers say the findings quantify the effects of ride sharing on the urban environment and offer guidance toward the design of efficient mobility systems.
This work was supported in part by grants from the National Science Foundation.
Media Contact
Get Cornell news delivered right to your inbox.
Subscribe