Scientists find 'holy grail' of evolving modular networks
By Anne Ju
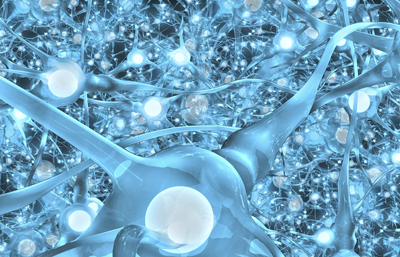
Many biological entities, from brains to gene regulatory networks, are organized into modules – dense clusters of interconnected parts within a complex network. Engineers also use modular designs, which is why a car has separate parts, from mufflers to spark plugs, rather than being one entangled monolith.
Modularity is beneficial, but how did it arise? For decades biologists have tried to understand why humans, animals, bacteria and other organisms evolved this way – what’s the evolutionary pressure to form modular networks, and how can engineers harness that knowledge for artificial intelligence? It’s a central biological question, and Cornell researchers think they’ve finally cracked it.
Modularity evolved, they say, as a byproduct of selection to reduce the “costs” of building and maintaining networks, which is accomplished by reducing network “wiring”: the number and length of network connections.
To test the theory, the researchers simulated 25,000 generations of evolution in a computer with and without a cost for network connections. “Once you add a cost for wiring, modules immediately appear. Without a cost, modules never form. The effect is quite dramatic,” said Jeff Clune, former visiting scientist at Cornell and assistant professor of computer science at the University of Wyoming.
The theory is detailed in a Jan. 30 publication in Proceedings of the Royal Society B <http://rspb.royalsocietypublishing.org/content/280/1755/20122863.short?…; by Clune; Hod Lipson, associate professor of mechanical and aerospace engineering and of computer science at Cornell; and Jean-Baptiste Mouret, a robotics and computer science professor at Université Pierre et Marie Curie in Paris.
The results may help explain the near-universal presence of modularity in biological networks as diverse as neural networks (i.e., animal brains), vascular networks, gene regulatory networks, protein-protein interaction networks, metabolic networks and, possibly, even human-constructed networks such as the Internet.
The results also have significant implications for fields that harness evolution for engineering purposes, collectively known as evolutionary computation. Trying to figure out how to evolve modularity has been one of the “holy grails” of these fields, Clune said. He hopes the finding will be especially helpful in work evolving robot “brains” – neural networks that can control robots – to enable them to begin to acquire the intelligence and grace of natural animals, such as gazelles and humans.
“Being able to evolve modularity will enable us to create more complex, sophisticated computational brains,” Clune said.
Clune’s dual background in biology and computer science led him to this work. Nearly 10 years ago, he met Lipson at a conference on evolving artificial intelligence. Lipson told Clune that he believed the key to their field was understanding how to evolve modularity – “I’m betting my career on it,” Lipson said.
Clune decided to join Lipson’s research group at Cornell to work on the problem, although it would take him nearly a decade to arrive on campus and get to work. Mouret flew in from Paris to join the team, and a summer later they had their answer.
“We’ve had various attempts to try to crack the modularity question in lots of different ways,” Lipson said. “This one by far is the simplest and most elegant.”
The work was supported by the National Science Foundation and the French National Research Agency.
For more information, visit <http://jeffclune.com/modularity.html> or email Jeff Clune at <jclune@uwyo.edu>.
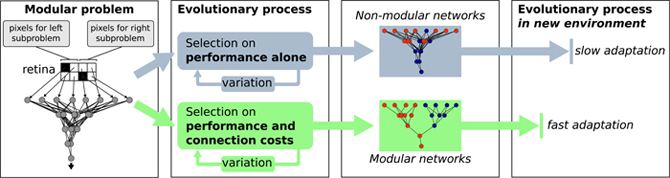
Media Contact
Get Cornell news delivered right to your inbox.
Subscribe