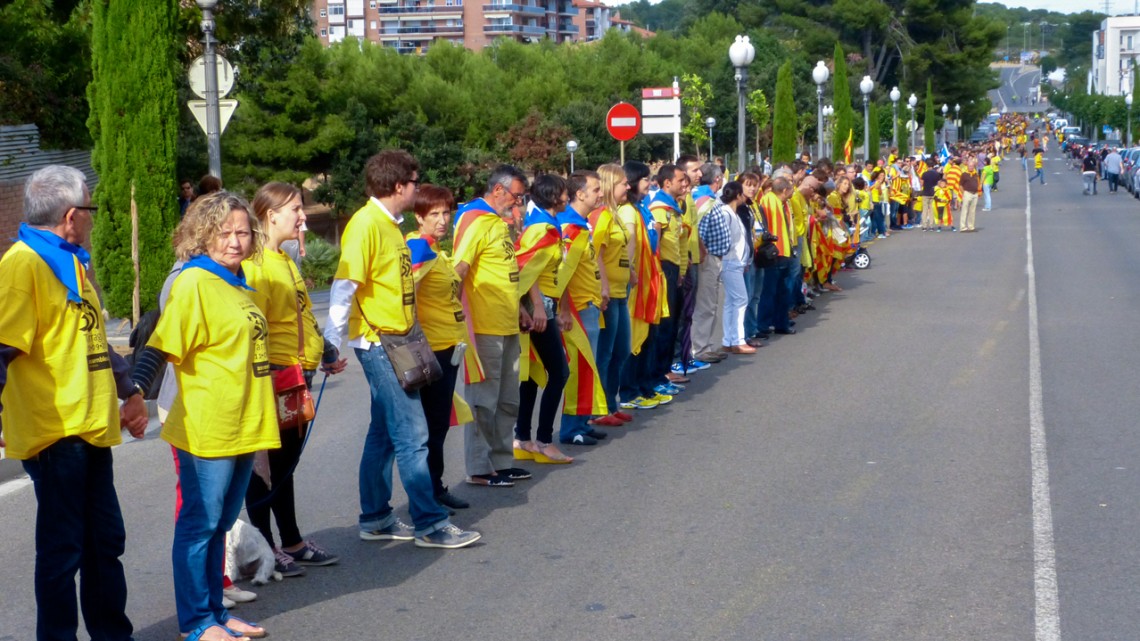
The September 2013 Catalan Way, in which 1.2 million people – many clad in yellow shirts and blue scarves – linked arms to support Catalan independence from Spain.
AI tool detects global fashion trends
By Melanie Lefkowitz
On the king’s birthday in Thailand – celebrated as Father’s Day – people often wear yellow shirts emblazoned with the word “DAD.”
On FreakNight in Seattle – a dance music event held around Halloween – revelers tend to wear sleeveless shirts, despite cool weather.
And in September 2013, 1.2 million people – many clad in yellow shirts and blue scarves – linked arms to support Catalan independence from Spain.
These are among the global insights gleaned from GeoStyle, a new artificial intelligence tool developed by Cornell researchers that scans millions of publicly available photos to effectively identify fashion trends around the world, as well as traditions and events with signature styles.
“A lot of people are continually uploading photos of themselves on the internet, because they want to share their style with their friends and the rest of the planet,” said Kavita Bala, professor and chair of computer science and senior author of “GeoStyle: Discovering Fashion Trends and Events,” presented at the International Conference on Computer Vision, Oct. 27 to Nov. 2 in Seoul, South Korea.
“When you’re looking at these large collections of images, there are many, many things you can do to understand how people live,” Bala said. “So we started off with the idea of looking at how people dress in different parts of the world: What are the commonalities, and what is distinctive to different areas? If anthropologists could see this record 100 years from now, they would understand a lot about our time just by looking at these images and getting insights from them.”
GeoStyle analyzes public Instagram and Flickr photos to map trends using computer vision and neural networks, a kind of artificial intelligence often used to sort images. Its models help researchers understand existing trends in specific cities and around the world over time, and its trend forecasts are up to 20% more accurate than previous methods.
For example, GeoStyle shows that year by year, more people wear black, but fewer people wear black in the summer than in the winter. The researchers also created a visualizer that allows users to view the popularity of a certain attribute – such as a pattern, hat or color – by city, over time.
To refine the avalanche of data GeoStyle generates, the paper’s first author, Utkarsh Mall, a doctoral student in computer science, developed a framework to automatically identify spikes – short-term changes, some annual and some occurring once – that buck the longer-term trends.
“We have all this cool machine learning technology that we’ve come up with to recognize images, but how do we make it useful?” said co-author Bharath Hariharan, assistant professor of computer science. “Our key question was, can we use this tool to automatically surface something we, as creators of this system, didn’t know before?”
In fact, the model was able to identify dozens of short-term style changes corresponding to events around the world, including many the researchers weren’t aware existed, such as Songkran in Bangkok, a festival celebrated in April on the Thai New Year.
Once it identifies a spike, the tool employs a text analysis based on photo captions to figure out what it might mean. The researchers at first thought that the spike in sleeveless shirts in Seattle had to do with Halloween, because it occurs around that time, but the text associated with the photos contained the word “Freaknight,” which helped them identify it as a distinct celebration.
“This was an example where analyzing the text really made a difference,” Hariharan said.
The project builds on StreetStyle, launched in 2017 by Bala and GeoStyle co-authors Noah Snavely, associate professor of computer science at Cornell Tech, and Kevin Matzen, Ph.D. ’15, of Facebook. StreetStyle detects trends based on time and location by analyzing millions of images.
The team is currently working with Denise Green, assistant professor of fiber science and apparel design, and other fashion experts at the College of Human Ecology, to improve their model. The tool can do a better job spotting trends if it knows what it’s looking for, Bala said.
“An expert can identify important visual features in a very different way than we can just by mining it,” she said. For example, she said, a student pointed out that the data showed the evolution of trucker hats from an accessory worn by farmers to one appearing on fashion runways to widespread popularity.
“One of our follow-ups from this work is improving the technology so that if you add a little expert information, you can improve the recognition and get an even finer-grained understanding,” Bala said.
Other potential applications for the technology include scanning satellite imagery to track changes in land use patterns, the researchers said.
The study was partly funded by the National Science Foundation and an Amazon Research Award.
Media Contact
Get Cornell news delivered right to your inbox.
Subscribe